Predictive maintenance (PdM) aims to predict when an asset might fail so that maintenance can be performed just in time to prevent the failure.
This approach relies on condition-monitoring equipment and advanced analytics to forecast the failure point.
Here’s a process for implementing predictive maintenance:
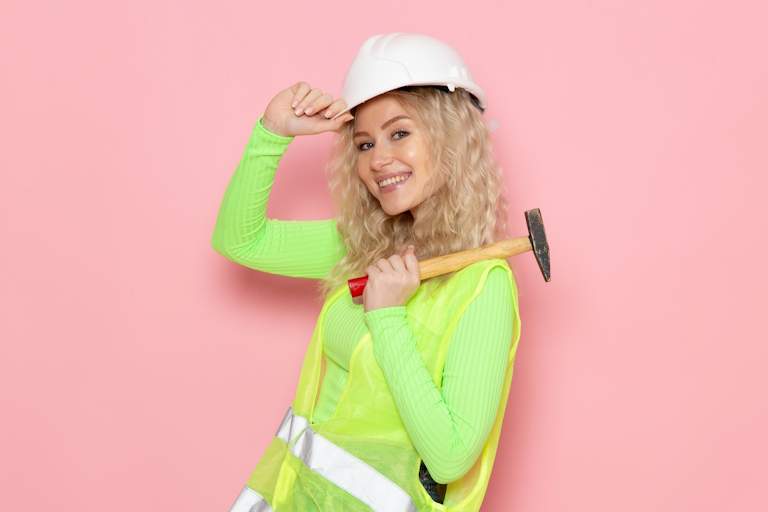
Data Collection
- Sensor Installation: Install sensors on critical assets to monitor various parameters such as vibration, temperature, pressure, and acoustic signals.
- Operational Data: Collect historical operational data, including usage patterns, environmental conditions, and maintenance history.
Data Integration
- Centralized System: Integrate data from various sources (sensors, historical records, IoT devices) into a centralized maintenance management system.
- Real-Time Data Streaming: Ensure continuous real-time data streaming to capture the current state of assets.
Condition Monitoring
- Threshold Setting: Establish baseline thresholds for normal operating conditions based on manufacturer guidelines and historical performance data.
- Continuous Monitoring: Continuously monitor the condition of assets in real-time, comparing current data against the established thresholds.
Data Analysis
- Trend Analysis: Analyze data trends to identify patterns and deviations that indicate potential issues.
- Machine Learning Models: Develop and train machine learning models using historical data to predict future asset behavior and failure points.
Failure Prediction
- Predictive Algorithms: Use predictive algorithms to assess the probability of failure within a specific timeframe.
- Remaining Useful Life: Calculate the Remaining Useful Life of the asset to determine how much longer it can operate before maintenance is required.
Maintenance Scheduling and Optimization
- Just-in-Time Maintenance: Schedule maintenance activities based on the predicted failure times to perform them just before the asset is expected to fail.
- Feedback Loop: Use post-maintenance data to update predictive models and improve accuracy. Analyze the effectiveness of the maintenance actions and refine strategies accordingly.
Continuous Improvement
- Regular Review: Regularly review and update predictive maintenance models and processes based on new data and insights.
- Technology Upgrades: Keep up with advancements in sensor technology, data analytics, and machine learning to enhance predictive maintenance capabilities.
By following this process, hotels can effectively implement predictive maintenance to foresee and prevent asset failures, reducing downtime, extending asset lifespan, and optimizing resources.
Interested in learning more?
Here are some great references to explore.
- ISO 17359: “Condition monitoring and diagnostics of machines – General guidelines.” This standard outlines general guidelines for condition monitoring and diagnostics of machines, which is a core component of predictive maintenance.
- “Maintenance Engineering Handbook” by Keith Mobley. This handbook provides comprehensive information on maintenance strategies, including predictive maintenance.
- “A Review on Maintenance Techniques for Industrial Equipment and its Machine Learning Algorithms” by Ulaganathan J and Sadyojatha K M published in the International Journal of Advanced Research in Engineering and Technology (IJARET)
About Q2
For over 25 years Q2 have helped hotels implement effective hotel operations solutions leading to improved guest satisfaction including engineering, planned maintenance, asset management, inventory management, and guest services. Sidekick and ESCAP cloud software platforms ensure that these plans are well-designed and executed empowering hotels to deliver memorable experiences to their guests.